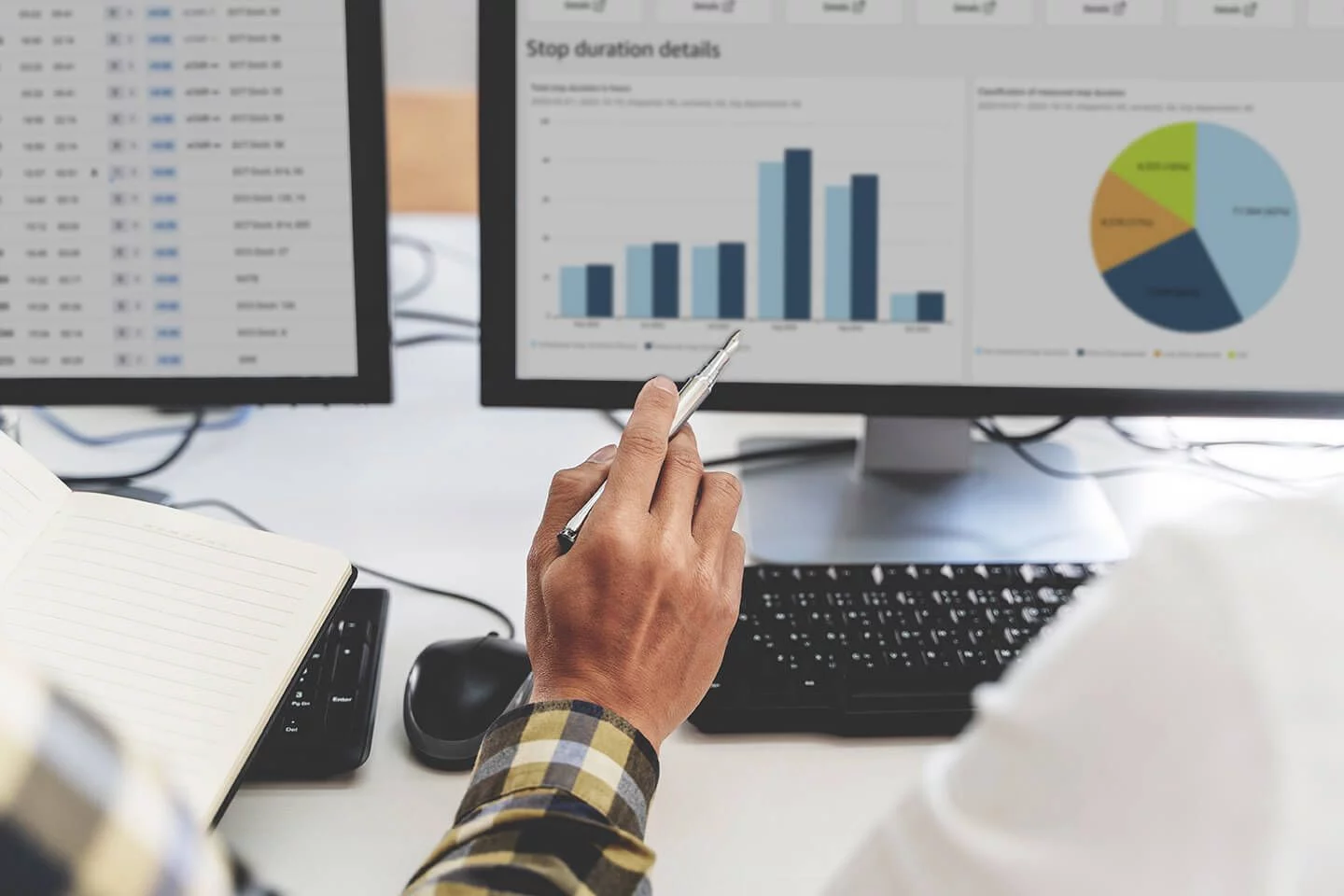
Data for Logistics: Why data quality matters and how Simacan enhances insights
What data does Simacan process?
Raw data alone has little value unless it is structured and analyzed properly. The Simacan platform processes and enhances data by linking multiple sources, including planning schedules, real-time traffic information, and realization data. In addition, artificial intelligence (AI) is used to generate new insights such as optimal routes with estimated distances and driving times, expected arrival times (ETA), and accurate arrival and departure timestamps.
By leveraging these datasets, Simacan provides actionable insights at every stage of transport, including pre-trip planning, real-time on-trip monitoring, and post-trip performance analysis. This blog focuses on how Simacan enhances on-trip monitoring and post-trip analytics to improve logistics operations.
On-trip and post-trip data insights
Simacan provides access to post-trip data through various channels. The Realised Trip Service allows users to retrieve raw trip data for independent analysis. The platform also includes a performance dashboard that offers insights into operational efficiency, including timeliness, planning accuracy, and data completeness. Users can export trip data for further analysis with external tools. Additionally, daily quality reports provide transparency into potential gaps in real-time data. Historical trip data is available at the stop level, enabling deeper benchmarking and trend analysis.
While users have the flexibility to analyze data independently, Simacan’s ready-to-use dashboards simplify the process, making insights more accessible without requiring extensive technical expertise.
Post-trip data can be obtained in several ways:
- Raw data at trip level: With Simacan’s Realised Trip Service (our push service), almost all received and generated data can be obtained. More information >
- Dashboard with insights of a transport operation like operation size, timeliness, planning accuracy and data completeness. Trip data can also be downloaded, to do further analysis with other analytical tools. More information >
- Quality reporting per trip at daily level: Provides insights into the reasons for limited real-time data per trip. More information >
- Historical trip data: Trip data at stop level, available for download. More information >
The importance of data quality
For data to be useful in analytics, it must be complete, timely, and reliable. The Simacan platform carefully filters out incorrect, missing, or outdated data to ensure high-quality insights. A common challenge in logistics data is the discrepancy between planned and actual trip execution. Sometimes, planned stop sequences do not align with reality, as vehicles may not reach all scheduled locations or may visit unplanned stops. Post-trip modifications can further complicate data accuracy, making it difficult to determine which version of the information is correct.
Realization data includes GPS coordinates that indicate where a vehicle is at a given time. However, if GPS signals are weak or delayed, they may inaccurately reflect a vehicle’s location, creating uncertainty about when a vehicle actually arrived or departed from a stop. When such inconsistencies occur, the Simacan platform makes estimates based on a combination of historical and real-time data.
Discrepancies in recorded timestamps can also arise due to unreliable planning or real-time data errors. For instance, if a vehicle is registered as having departed when it has actually remained stationary for several minutes, the system may record incorrect stop and travel durations. When significant inconsistencies are detected, Simacan filters them out to maintain data integrity.
Some trip phases are inherently less reliable than others. For example, when a vehicle starts a trip from a distribution center, it is difficult to determine the exact time it was first present at the location. Only the departure time is known with certainty. These nuances demonstrate the complexity of transport data, making rigorous data validation essential. By assessing the availability, completeness, and accuracy of each dataset, Simacan ensures that unreliable data is removed, leaving a clean and usable dataset for analytics.
- Should manually cancelled trips be included in the invoicing? What about trips which were not cancelled but it remains unclear whether they were carried out or not?
- Has a vehicle arrived on time at a location if we have defined 15:00 as an ‘on time’ value, but we know it was not there at 14:55 but it was at 15:05?
In the platform, the arrival time is the first GPS coordinate measured at the specified location. But with a low update rate of GPS coordinates, the vehicle might have arrived earlier. - Has a vehicle arrived on time at a location if we have defined 15:00 as an ‘on time’ value, but is measured to be near the location at 15:00?
In the platform the arrival time is the first GPS coordinate measured is near / at the specified location. - The average dwell or stop time for a location is determined from the historically measured dwell times. Suppose a driver regularly takes his lunch breaks at a certain location, is it desirable for these breaks to be included in determining the average dwell time of that location? And is it a problem if a dwell time is included in a calculation while the measurement deviates 30 minutes from reality?
- How can it be evaluated whether the planned driving time is accurate if the measured driving time includes dwell and break times? While planned driving times do not include these?
- Etcetera…
Why data analytics is essential for logistics
The logistics industry is shifting from simply gaining real-time visibility to actively optimizing transport efficiency and sustainability. High-quality data helps businesses make better decisions, reduce costs, and enhance service reliability. Companies can use transport data to improve operational timeliness, streamline invoicing, reduce unnecessary dwell times, and quickly address inefficiencies in their supply chain.
Data-driven decision-making enables companies to become more agile and responsive to market demands. By analyzing trends and performance metrics, organizations can fine-tune their logistics strategies, improving overall efficiency and customer satisfaction.
Challenges in logistics data analytics
Another frequent issue arises in defining on-time arrivals. If an arrival time of 15:00 is considered punctual, but the vehicle was last recorded at a nearby location at 14:55 and then again at 15:05, should it be classified as on time? GPS update intervals can sometimes create gaps in the data, making precise arrival time calculations challenging. Similarly, determining whether a stop duration is valid can be complex. If a driver consistently takes lunch breaks at a specific location, should these breaks be included when calculating average dwell time? These decisions impact how data is interpreted and utilized in operations.
Each transport organization establishes its own data thresholds based on its operational requirements. Simacan helps businesses set the right parameters and data definitions to ensure consistency and avoid misinterpretations.
Why choose Simacan’s ready-made dashboards instead of manual analysis?
The Simacan platform simplifies this process by offering ready-made dashboards that provide structured insights without requiring manual data handling. Users can quickly identify trends, evaluate performance, and make data-driven decisions without needing advanced technical skills. This saves time, effort, and costs, allowing logistics managers to focus on optimizing their operations rather than spending hours processing raw data.
Unlock the power of your logistics data
You may also like:
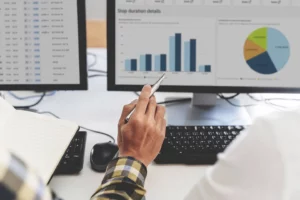
Transport Performance Monitor
Simacan’s Transport Performance Monitor delivers real-time insights to optimize fleet efficiency, track trends, and improve logistics. ISO 27001-certified, it ensures secure, data-driven decisions for resilient and collaborative supply chain operations.
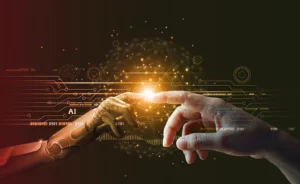
100% Digital logistics: possible?
100% Digital logistics; is fully automated handling of transport orders possible? What should you consider when you start digitalising your transport processes?